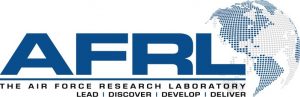
The Air Force Research Laboratory (AFRL) and QC Ware are exploring the use of one of QC Ware’s proprietary quantum machine learning algorithms to understand the purpose or mission objective of unmanned aircraft by observing its flight path, according to a company news release. Called q-means, the quantum algorithm for clustering and classification can also be applied to a variety of AFRL mission applications.
QC Ware has been an early partner in advancing a key AFRL objective — supporting quantum algorithm development in optimization, machine learning, and quantum simulation for implementation in near-term quantum computers. The project is part of a larger AFRL effort to engage with expert researchers in industry, academia, and the Department of Defense to apply quantum information science to Air Force and Space Force concerns and ensure they remain the most advanced and capable force in the world.
“AFRL is pleased to partner with QC Ware on the development of quantum machine learning algorithms. Early and continued investment in quantum software firmly aligns with AFRL’s quantum strategy. As quantum computing hardware continues to rapidly advance and become more practical, we believe that these types of algorithms will readily find applications to real-world Air Force scenarios,” said Mike Hayduk, Deputy Director, AFRL Information Directorate.
“QC Ware values our long-term partnership with AFRL. We believe quantum software is a mission-critical advantage for organizations and nations, and can be useful in the near-term,” said Matt Johnson, CEO, QC Ware. “QC Ware’s research leadership in machine learning algorithms supports AFRL’s exploration into how quantum applications can ensure U.S. defense and space organizations maintain their superior edge.”

In the project’s first phase, AFRL and QC Ware researchers identified use cases for testing and evaluating the q-means algorithm to classify the flight path behavior of unmanned aircraft. It focused on designing and delivering software for a prototype q-means algorithm for clustering and classification that could be executed on commercially available quantum computers and quantum simulators, and would have the potential to provide improved performance over classical algorithms for a broad variety of other Air Force missions as well. The team used standard software and libraries in building the q-means software to enable AFRL researchers to run and modify the software themselves.
q-means is significantly faster than its classical counterpart, k-means. Both algorithms use the same technique in clustering similar data points together, and are especially useful when data scientists are trying to better understand similarities of items in their data set.
Compared with k-means, the q-means approach to machine learning scales much better with more complex, high-dimensional data sets. The greater the number of dimensions the finer the granularity, enabling richer tracking of flight paths and other behaviors. The q-means method is 10x faster than k-means when clustering data sets with 64 dimensions, 30x faster on 256 dimensions, and 100x faster on 1,000 dimensions.
In the project’s second phase, the research collaboration will focus on evaluating the performance of the algorithm, using real data and implementing the algorithm on currently available quantum hardware. The experiment’s objective is to determine how much quantum resources are needed in terms of qubit count and error rate to achieve performance speed-ups over classical algorithms for clustering and classification.
“AFRL’s ongoing investment in algorithm development and in benchmarking the performance of quantum machine learning algorithms over classical algorithms, using real data and real quantum hardware, is encouraging to quantum machine learning research efforts,” said Iordanis Kerenidis, Head of Quantum Algorithms-International, QC Ware. “We view our research collaboration with AFRL as an important opportunity to implement QC Ware’s landmark q-means algorithm to solve computational bottlenecks in key mission applications.”
About AFRL
The Air Force Research Laboratory (AFRL) is the primary scientific research and development center for the Department of the Air Force. AFRL plays an integral role in leading the discovery, development and integration of affordable warfighting technologies for our air, space and cyberspace force. With a workforce of more than 11,000 across nine technology areas and 40 other operations across the globe, AFRL provides a diverse portfolio of science and technology ranging from fundamental to advanced research and technology development. For more information, visit: www.afresearchlab.com.
About QC Ware
QC Ware is a leading quantum-as-a-service company focused on the development of applications for near-term quantum computing hardware. With a team composed of some of the industry’s foremost experts in quantum computing, QC Ware is growing rapidly and generating substantial revenue from global enterprise and government sector customers including the Air Force Research Laboratory, Aisin Group, Airbus, BMW Group, Equinor, Goldman Sachs, and Total. QC Ware Forge, the company’s flagship quantum computing cloud service, is built for data scientists with no quantum computing background. It provides unique, performant, turnkey implementations of quantum computing algorithms. QC Ware is headquartered in Palo Alto, California, and supports its European customers through its subsidiary in Paris. QC Ware also organizes Q2B, the largest annual gathering of the international quantum computing community.
If you found this article to be informative, you can explore more current quantum news here, exclusives, interviews, and podcasts.