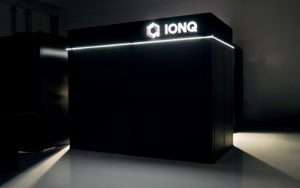
QC Ware and IonQ report they teamed up to run a machine learning algorithm for classification on an 11-qubit quantum qubit.
The experiment is discussed in QC Ware’s Medium blog and reported in a paper on arXiv, a pre-print depository.
According to the post, the teams focused on loading classical data onto quantum states in a resilient way to facilitate quantum machine learning — or QML — applications.
Team members reported: “Central to the success of our experiment are QC Ware’s own Forge Data Loader™ technology, which optimally transforms classical data onto quantum states, and the high-quality, fully connected qubits of IonQ’s 11 qubit system.”
According to the researchers, loading classical data onto quantum states is challenging for a number of reasons. First, while most experts assume that QRAM — quantum random access memory — must be available, most proposals require significant hardware investments, qubit count and circuit depth. The team reports that the Forge Data Loader provides a better alternative to QRAM. The technology can load such data points with just 100 qubits and a circuit depth of 100, the researchers added.

The team reports their results: “QC Ware’s quantum algorithm running on IonQ’s hardware performed at the same
level as the corresponding classical algorithm, identifying the right digits 8 out of 10 times on average, the same number of times as the classical algorithm running on classical hardware. This can be seen below in the classical and quantum confusion graphs for the MNIST datasets. This is the first time ever that a classification task with 10 classes has been performed on a quantum computer.”
This technology offers several long-term advantages in useful quantum computing, according to the researchers.
First, it takes fewer steps and less time to complete the calculations, meaning processing is faster and better. Second, the team argues that this method is scalable. Accuracy of the algorithm should hold as the problem size increases without the need for error-corrected qubits.
The advance could have the following industry impacts, according to the researchers:
- Opens the way for various quantum machine learning applications, including natural language processing, decision-making, customer recommendations, and fraud detection
- Speeds up the industry timeline for practical QML applications on near-term quantum computers
- Shows the potential for the coming generation of quantum machines to outperform classical computers
The teams include: Iordanis Kerenidis, head of Quantum Algorithms — International at QC Ware, and Sonika Johri, Senior Quantum Applications Research Scientist at IonQ. They announced their findings at Q2B20.
For more market insights, check out our latest quantum computing news here.