*Originally published on the Airbus website
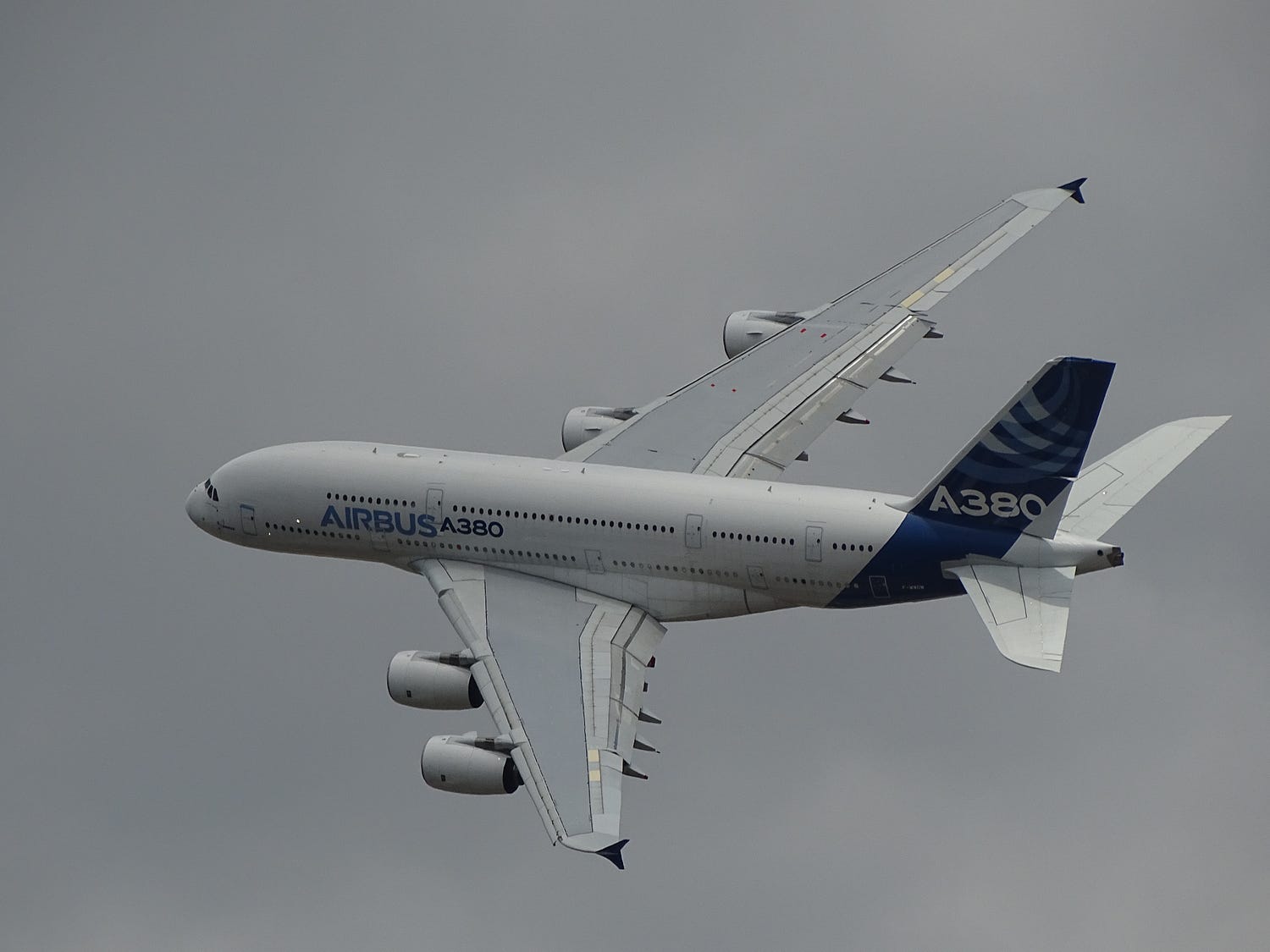
The European concern Airbus, one of the giants of the aerospace industry — which launched a global quantum computing competition called The Airbus Quantum Computing Challenge in January 2019 — has just released its five shortlisted finalists for the competition. From an admission list of 36 entrants, the lucky winners will be given ‘unique access to resources and the chance to work collaboratively with Airbus’ experts from the flight physics sector’. The winner is to be announced in December 2020.
With the intention of ‘bringing flight physics into the Quantum Era’ by way of teaming up Airbus’ top experts from engineering and flight physics with top academic and industry talent in QC, the Challenge will evaluate exciting proposals submitted to them.
This year’s five finalists are:
Team Capgemini*
The design, development, and production of a new aircraft model takes several years and is dependent on trade-offs between many economic factors.

In the quantum Airbus challenge we propose two novel algorithms inspired by HHL’s well-known algorithm for matrix inversion and QSVM. Both algorithms are designed for an order of magnitude performance gains by reducing parameter space in wing box optimization.
The algorithms are applicable for LSFT, and NISQ quantum computers respectively, therefore, bridging the gap until the technology has matured. The work is a close collaboration between Capgemini’s aviation HPC, and quantum technology team, thereby acknowledging the need for end-to-end know-how for the design and implementation of quantum algorithms.
Although it is currently impossible to perform a full aircraft optimization, due to the complex interaction between components, the proposed submission may ultimately pave the way for multi-modal, full aircraft optimization.
Team Machine Learning Reply*
In this submission, we solve the loading optimization problem of the Airbus Quantum Computing Challenge using a quantum algorithm.
Finding the optimal loading for a plane is a challenging task for classical algorithms, especially because the solution must respect several flight constraints simultaneously. In this work we prove that optimization problems like this one can be mathematically modelled and solved through Quantum computing, offering a new solution path forward.
Team Niels Backfisch*
Several authors showed that averaging over n uncorrelated machine learning models reduces their combined error by a factor of n.
The proposed method computes the predictions of exponentially many quantum machine learning models, including all possible uncorrelated models, for the cost of a single model.
With some technical mathematical requirements, it even allows the training of such a model for regression. The training finishes with 99% probability within 10 epochs, regardless of the data or the model size.
Therefore the proposed method might decrease the machine learning model’s error by a large factor, growing exponentially with the number of qubits, while only needing to see the data 10 times or less.
Team Origin Quantum*
Computational Fluid Dynamics is important for its application in aircraft design. As the number of grid points grows, evermore powerful computing resources are needed. In our submission, we propose a quantum-classical hybrid method for accelerating SU2, an open-source software for CFD. Our method is based on the Finite Volume Method. We implement this method by replacing the space integration and the linear solver modules with quantum modules and preserve the iteration (time integration) process. The time complexity of our method is O(polylog N), poly-logarithmically dependent on the number of grid points. Our quantum algorithm is implemented in SU2 with submodules written by a quantum programming language QPanda. We assert that the fault-tolerant quantum computing and the quantum random access memory are necessary for showing the quantum advantage for this algorithm.
Team Universidad de Montevideo*
The problem of determining the optimal loading strategy for packing merchandise in vehicles has been addressed using different techniques like dynamic or genetic programming. Inspired by the well-known Knapsack Problem, our solution aims at determining the optimal configuration of maximizing the loading of a cargo airplane -subject to constraints- using quantum algorithms.
Our approach is based on the Variational Quantum Eigensolver (VQE) algorithm. Understanding the complexity of classical computational methods we aim to find the quantum advantage provided by the VQE in a hybrid solution.
As the number of packages to be transported grows the number of possible combinations increases posing a serious challenge to be solved by classical means.
Based on the complexity order of the problem we project the number of necessary qubits to solve it and the feasibility of running the algorithm in a quantum computer currently available or in the near future.

The five teams, all top experts in their respective fields, hope to gain experience and learn from the world-class facilities at Airbus.
About the competition*
With traditional computers gradually approaching their limits, the quantum computer promises to deliver a new level of computational power. As an active user of advanced computing solutions, Airbus is at the forefront of a paradigm shift in the computing world exploring how quantum computing could solve key questions for the aerospace industry, and forever alter how aircraft are built and flown.
To take us one step further, Airbus launched a global quantum computing competition in January 2019, challenging experts and enthusiasts in the field to join forces with the company for a Quantum Era in aerospace.
The Airbus Quantum Computing Challenge (AQCC) addresses aerospace flight physics problems developed by company experts. Airbus is providing the quantum computing community with a unique opportunity to test and assess the newly-available computing capabilities to solve some of our most difficult and complex problems, and in doing so, further legitimise and fuel progress of this technology.
The challenge puts forward five distinct flight physics problems with varying degrees of complexity, ranging from a simple mathematical question to a global flight physics problem.
It is open to the whole scientific community of experts, researchers, start-ups, academics and will lay the ground for the ultimate shift to a Quantum era in aerospace.
If you found this article to be informative, you can explore more current quantum news here, exclusives, interviews, and podcasts.